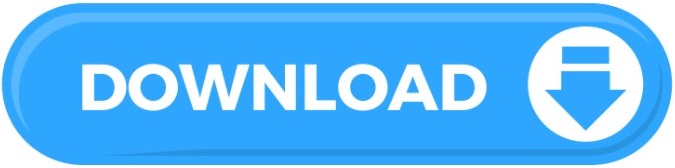
See here for more on Type S and Type M errors.ĭoes this matter? If we just do straight Bayesian inference with continuous prior distributions and work with posterior inferences, then it’s not really so important. The well-known problem of publication bias could lead to systematic Type M errors, with large-magnitude findings more likely to be reported. I make a Type M error by claiming with confidence that theta is small in magnitude when it is in fact large, or by claiming with confidence that theta is large in magnitude when it is in fact small. 2 and is statistically significantly different from zero, then our scientific claim is that theta is positive, not simply that it’s nonzero.Ī Type M error is an error of magnitude. I think it’s fair to say that classical 2-sided hypothesis testing fits this framework: for example, if our 95% interval for theta is, or if we say that theta.hat =. I make a Type S error by claiming with confidence that theta is positive when it is, in fact, negative, or by claiming with confidence that theta is negative when it is, in fact, positive. In any given study, there might be many thetas of interest.)Ī Type S error is an error of sign. (For example, theta could be a regression coefficient, or a comparison between two treatment effects. In the applications I’ve worked on, in social science and public health, I’ve never come across a null hypothesis that could actually be true, or a parameter that could actually be zero.Ī Type 2 error occurs only if I claim that the null hypothesis is true, and I would certainly not do that, given my statement above!īut I certainly have made errors! How can they be classified? For simplicity, let’s suppose we’re considering parameters theta, for which the “null hypothesis” is that theta=0. How can this be?Ī Type 1 error occurs only if the null hypothesis is true (typically if a certain parameter, or difference in parameters, equals zero). I’ve never in my professional life made a Type I error or a Type II error.
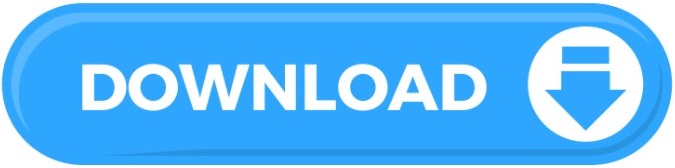